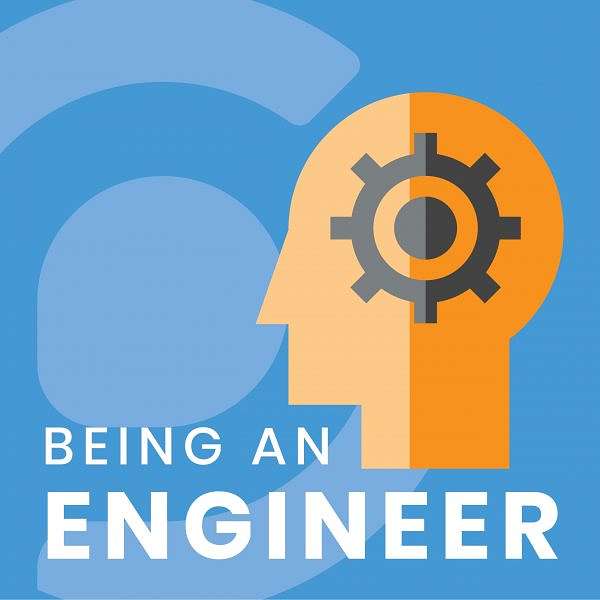
Being an Engineer
Being an Engineer
S5E48 Kordel France | Olfactory AI
Today, we are thrilled to host an episode with an exceptional guest pushing the boundaries of artificial intelligence. Kordel France discussed the challenges and potential of digitizing scents. He highlighted the need for a standard data format for olfactory AI and the complexities of detecting and analyzing air molecules. Kordel emphasized the importance of olfactory AI in various industries, including automotive for HVAC systems and quality control, and aerospace for detecting chemical leaks. He predicted that reliable olfactory AI for consumer products is 10 years away, while navigation and aerospace applications could be achieved sooner. France also stressed the need for better integration of AI with hardware and the importance of team collaboration in engineering.
Main Topics Covered:
- Kordel's background and early interest in engineering
- The concept of olfactory AI and the lack of research in this area
- Current state and future potential of olfactory AI technology
- Applications of olfactory AI in the automotive industry
- Challenges in developing olfactory AI systems
- Integrating AI with mechanical systems
- The future of autonomous vehicles
- Advice for young engineers
About the guest: Kordel France, an AI architect and technologist, is at the forefront of developing machine olfaction—teaching robots to smell. With a career spanning roles from Chief Technology Officer at startups to his current work at Toyota, Kordel's expertise integrates computer vision, sensor systems, and multi-agent learning.
Links:
Kordel France - LinkedIn
🚀 Join Us at PDX 2025! 🚀
PDX 2025 is the Product Development Expo designed for engineers who want hands-on training from industry experts. PDX focuses on practical skill-building, cutting-edge tools, and real-world solutions.
📅 October 21-22, 2025
📍 Mesa Convention Center, AZ
🔗 https://reg.eventmobi.com/product-development-expo-2025
About Being An Engineer
The Being An Engineer podcast is a repository for industry knowledge and a tool through which engineers learn about and connect with relevant companies, technologies, people resources, and opportunities. We feature successful mechanical engineers and interview engineers who are passionate about their work and who made a great impact on the engineering community.
The Being An Engineer podcast is brought to you by Pipeline Design & Engineering. Pipeline partners with medical & other device engineering teams who need turnkey equipment such as cycle test machines, custom test fixtures, automation equipment, assembly jigs, inspection stations and more. You can find us on the web at www.teampipeline.us
How do you digitize a scent? Right? What's the data standard for scent images or PNGs and JPEGs, audio files or wave forms and spectrograms? But what is a smell? There's not even agreed upon data standard.
Aaron Moncur:Hello and welcome to the being an engineer podcast today, we're thrilled to host an episode with an exceptional guest pushing the boundaries of artificial intelligence. Kordel France, an AI architect and technologist, is at the forefront of developing machine olfaction, teaching robots to smell with a career spanning roles from Chief Technology Officer at startups to his current work at Toyota. Kordels expertise integrates computer vision sensor systems and multi agent learning. Kordel, thank you so much for joining us on the show today.
Kordel France:Aaron, thank you for having me and thank you to your guests. Your guests.
Aaron Moncur:All right. Can you tell us a little bit about your your background? You know? How did you get from at whatever age you started becoming interested in engineering and AI, to where you are now?
Kordel France:Why? Man, so the age that it started for me was actually is about three or four years old. So I grew up on a farm, and my father had a bunch of these agricultural tractors, and he put some GPS guided software on it one day, and the allowed the machine to drive itself. And as a toddler, you're seeing this giant machine drive itself with a steering wheel turning, and it needs no human interaction. It was something straight out of a sci fi movie for me. And so from that day forward, I always ask my dad, I go, how do I work on this? How I build these things? Right? Like, it's like a legitimate robot. And he always goes, you gotta be good at math. You gotta go be good at, you know, physics and engineering and chemistry and science and going to these fields, and that really catapulted my career from there was that those particular moments on the farm and seeing me self driving tractors, and then my father just kind of constantly beating it in my head that you've got to be good the engineering disciplines. And so I've had an infatuation with this since I was literally a toddler.
Aaron Moncur:That's amazing. I mean, most people, they're lucky if they know what they want to do by the time they graduate college, and you knew when you were three or four years old. So I've heard so many great stories about folks who grew up on a farm becoming engineers. It seems like there's some magic there, like the experience that you have growing up on a farm just gives you, inherently, some tools as an engineer that that the rest of us who haven't grown up on farms don't have it. Is there something to that, or is it just a wild coincidence? I definitely
Kordel France:think there's something to it. Because growing up on a farm, you're exposed to a lot of mechanical engineering and electrical engineering, there's always something to build. There's always something that breaks that you've got to fix, and you're always limited with resources. Everything you need is never right there. So you have to get invented, and a lot of times that tools or you have to go fetch parts. And so you get used to supply chain issues and working under a deadline and trying to make sure that you beat the weather. So working, you know, under under very aggressive deadlines that you can't control with the weather and with modern machinery on in agriculture, there's a lot of software in it now, so you get exposure to really mechanical engineering and software or mechanical electrical and software engineering disciplines by just being exposed to agriculture in the farm, and so I definitely think there's something to it that kind of manifests that engineering talent, if it's buried deep inside you by working on a farm or growing up around it,
Aaron Moncur:yeah, yeah. I love that you brought up how you have deadlines that are controlled, sometimes by the weather, right outside of your control, so you have to learn how to work quickly. Yeah, what? There should be some kind of a training ground, like a new school, where, if you know you want to become an engineer, you don't go to college, you go to a farm. And that's your hands on real world practice. I would
Kordel France:definitely attest to that it teaches you a lot of discipline that you won't get it elsewhere. And, yeah, it's, it's, it's it's really a crash course on several different modes of product development. The products you're building on a farm is the produce that you're, that you're that you're chilling, and that you're growing every day. And it's, I mean, there's a product development life cycle that lasts a year, there's seasons, there's limited parts, there's demand for parts. It's, it's, it's really a good pseudo product development simulation, if you will.
Aaron Moncur:Yeah, yeah, that's terrific. All right. Well, new business idea for for next year maybe. Okay, let's, let's talk about AI a little bit. So you are, I know you're a very humble individual, and maybe you wouldn't refer to. Yourself as an expert, but based on what I've seen, you are an expert in AI, and specifically, you have this really interesting niche within AI of olfactory AI. How did you get interested in that? So
Kordel France:I got interested in it probably about seven or eight years ago. It was it was late. It wasn't something that I originally thought of. I mean, you think of you think of building olfaction, which is a fancy term for machine smell. You think of building that it's not something that's taught in school, it's not something that's referred to a lot. And so I wasn't exposed to it, but I got exposed to it with a project that I was actually contracted under, with a startup that I founded to build the AI for these sensors. And the sensors at the time were very rudimentary. They're detecting one compound. And I kind of became infatuated with the whole idea of being able to replicate the sense of smell machines. And if you look at all the other sensing modalities that humans have, vision, audio, being able to hear, being able to speak. And you look at the field of artificial intelligence, those sensing modalities have a ton of research innovation investment, but the sense of smell, I would argue, is very asymmetrical, and that it doesn't have that same sense, that same level of innovation investment in engineering. But it's not because of a lack of interest and or maybe a lack of potential, in my opinion, it's just that I don't think folks have been really exposed to all the potential applications out there. And so I kind of just started diving deeper and deeper into the field of olfaction and trying to figure out, why can I not put why don't why is there not a sensor that exists that can allow a robot to navigate the same efficacy that a dog can with with its nose, or a moth can and slay pheromones, right? Why are we not replicated that yet? And there are, there is a lot of evidence that that has been replicated, but it never really left the lab, and the reasons I found were that it's very difficult to accommodate, simulate and adjust for the environmental conditions, for air plumes and aerodynamics that you have to, you have to analyze in order to detect a chemical within the air. And if you break, if you break olfaction down to its like fundamental principles, you're really trying to touch air molecules. That's that's the fundamental, that's the fundamental way to think about it. And so how do I detect or touch all the chemicals around me? And there's several different technologies to do that. And so I just became obsessed with trying to understand, like, why? What? What's the state of the art? What's the best way to basically replicate a dog's nose or moth antenna or any other olfactory sensor? And there's, there's a lot of potential there that just is largely untapped, and we see a lot of investment right now in a lot of these other modalities, but, and there's a lot of competition as well, especially with research. I mean, there's new research papers published every day in vision, in large language models and everything going on, but you don't see as much competition in the sense of smell. And so if you kind of take a step back and consider all of that that I just said, it creates kind of a compelling opportunity to work on. There's less competition. There's a lot of potential. And I mean, there's so much data in the air around us that is not being really capitalized on to its full potential, and so that kind of just painted my trajectory from there.
Aaron Moncur:Cool. So where are we right now in the the technology surrounding olfaction, are we at a point where we can do most of what the world the markets want to do, or is there a pretty significant gap between current technology and where we kind of want or need to be? In
Kordel France:my opinion, there's a pretty significant gap between where we are and where we where we want to be. And I think a lot of that has to do to the fact that there's a lot of the applications, and the potential for machine o fraction is not really being popularized or considered. So there's a lot of investment in monetizing breath and trying to understand, like, all the chemicals in breath. So when you when you exhale, that's there's a ton of data within breath that gives you a status indication on how your body's doing, and you can detect several medical conditions that way. So there's a lot of investment in that space from the machine of action perspective. But for example, if you consider like virtual reality, augmented reality sense, vision, audio. So yeah, vision, audio and speech are integrated in augmented reality headsets, but you don't see sent there. Why is that? Well, it's, how do you, how do you digitize a scent? Right? What's the data standard for scent images or PNGs and JPEGs, audio files or wave forms and spectrograms? But what's, what is a smell? There's not even agreed upon data standard, so that's the first thing that needs to be so. World. But if you look at all these other applications, I mean space, they send these giant machines up to detect life on other planets or evidence of life on other planets, and they're huge, and those can be easily compressed and replicated by other technologies using olfaction. And the perfume industry has a huge need for olfactory sensors to do quality control on their perfume, colognes, etc, and food, food quality control, food contamination, agriculture. I mean, I could go, I could talk for four hours on all the different applications for olfaction, but there were not the technology is not quite there yet for it to be monetized in a consumer fashion, because a lot of the problems that have to be solved come back to the sensor level and being able to compensate for a lot of the adverse aerodynamics that you'll find that really just kind of paint chaos if you don't analyze them the right way.
Aaron Moncur:How far away do you think we are from achieving some minimum viable level of olfactory AI. Are we a year away? Are we 10 years away
Kordel France:to get it on, to get olfaction to the same level of magnitude or status as vision and hearing? I think we're probably 10 years away to get something into a consumer product where it's as reliable as, for example, like a smartphone camera or microphone, I think, I mean, we, you could argue we're already there in some aspects, but more some of the applications that I like to see, I think we're, we're probably five years away, and if I can help it, hopefully sooner.
Aaron Moncur:Excellent. Yeah, all right, so you're working at Toyota right now automotive, and I'm sure there's plenty we can't get into there because of confidentiality, so we'll keep this pretty general. Do you see olfactory or olfaction as part of the automotive industry? Or is your interest in olfaction kind of separate from your day job.
Kordel France:There's a lot of applications that olfaction can be applied to in the automotive industry. So they are they will merge eventually. My the disciplines right now are separated and that I don't use olfaction at my my job at Toyota, that's kind of separated into my PhD and my research there, and previous startups, but the application and automotive, I mean, anything with an HVAC system will use olfaction one day. And so being able to put those into automotive applications or automotive HVAC systems is given there, also on the manufacturing line, fuel leaks, quality control of parts. There's a lot that olfaction can be used for. One thing that I'm particularly excited about in the automotive realm, aside from just integrating them into the HVAC system. HVAC systems is for manufacturing and being able to detect chemical leaks and batteries. So there are a lot of chemicals that go into manufacturing batteries, right? That's, that's a well known, well known statement, but being able to quality control leaks that come out of these cells as you're building, you know, hundreds of lithium ion batteries. That's, that's something that could be addressed by olfaction, because you'll be able to spot it Whitaker, then you'll be able to examine it through vision or any other modality. So there's a lot of applications there. The other thing that I'm probably and this is the probably application I'm most passionate about, is being able to navigate by scent, and so being able to put these sensors onto a vehicle, whether it's a drone or an automobile or or or anything, being able to navigate by scent, just as a mothwood or a dogwood. I think that's, that's something that we'll, we'll see in the future as well, which is something I don't, I haven't seen that at all yet. Yeah,
Aaron Moncur:that's fascinating, thinking about the Boston Dynamics dogs, right? With some kind of Cordell invented olfaction, implemented into Absolutely, let's see. So your work at Toyota kind of focuses on generative AI. What if you could just discuss, kind of generally, what? Of course, there's like autonomous vehicles. That's That's what comes to mind first and foremost, when I think about AI and the automotive industry, are there other areas within automotive where where AI is starting to gain a foothold? There
Kordel France:are several instances within automotive where AI starting to get a foothold. You could argue almost every part of the vehicle is going to be touched at some point by by AI, you see a lot with the voice assistants that are coming out on vehicles these days. My particular focus and my role is on manufacturing, robotics and manufacturing and integrating artificial intelligence in the manufacturing. It's interesting because manufacturing is so complex, and you. Once they get to a process that is tried and true, they're very, I'd argue, they're very apprehensive to refine it or alter it, because there are always quotas you have to meet for vehicle, manufacture, any product, really, there's always quotas that have to be mad. They shareholders to appeal, and so you don't want to disrupt the process. And so while we have evidence that we could optimize manufacturing processes in some way, or the robotics on a manufacturing line, being able to find the the opportunity to test is a bit difficult, because you have to either have a very replicative environment to test on, or you have to stop the manufacturing line. And so that's that's one problem that's kind of lies beyond AI that I think any manufacturer is going to have to try to solve. But there is a lot of opportunity to optimize so many different parts of manufacturing, because when you go into high scale manufacturing, you're using a lot of robotics in pretty much any product. So when you and a lot of those, I'm I'm even apprehensive to say robotics, because they're really just automated machines reading a script, and if something goes wrong, they can't fix themselves. So that's where I think a lot of potential with AI right now lies in robots, is being able to do error correction and really understand, okay, I put the bolt in the wrong place. Where is the bolt? If I dropped it, can I pick it Can I pick it back up and put it in the correct place? And, you know, not let me reassess the situation and is it correct, being able to have that, that high level logic is something where I think AI and robot, robot robotics are really going to merge quite well to accelerate manufacturing scale even more,
Aaron Moncur:if you were to kind of part the curtains and shows behind the scenes. What AI looks like behind the scenes? What is that like, like? What are some of the tools that are commonly used to develop AI? Is it just this massive database? I mean, I'm familiar with the term neural network, but I admit I don't really know what that means. What does the technology look like it. It's like root level. If
Kordel France:you take AI down to its most fundamental level, it's all math and statistics, all of it. Now it's accelerated through computer science and software frameworks that basically allow those computations through math to happen very quickly or in parallel or simultaneously. But it's all math and statistics when you drill down to it. And with statistics comes a lot of data. The need for a lot of data. So you mentioned databases all AI models need data in order to make sense of the world and have some functional and positive outcome. One thing that I've been really interested in, and why I think machinofaction is quite an interesting problem is, is being able to make sense of less data. So it's not really a hard problem anymore to throw a bunch of data at a machine learning model, neural network, if you will, and get a good outcome from it, or to get the objective that you're trying to solve. If you throw a bunch of data at a model, it will learn something. Now the question is, is it going to learn the pattern? Do you want it to learn? Or is it is it going to uncover new patterns that you didn't see before, but are obvious to a mathematical model? But how do you go the other way? How do I take very minimal data or no data at all, and make sense of it? That's where machine ofaction is particularly interesting, because there's not a lot of data. There's not even agreed upon data standard, as I mentioned, and there's not a lot of data available in the world to train these giant machine learning models to make sense of smell. So you really you have to start at the most fundamental level and create a bunch of data, or you have to teach AI to learn from less if you work in machine learning, you know that you can build basically an object detection model, or image recognition model, speech recognition model, whatever. And you can go online and download a bunch of data that will help you get started. There are terabytes of publicly available data sets that are open source, that allow you to get started and build a machine learning model with those modalities. But it's not the case for scent, for the sense of smell. So we've, we've that's a that's a worthy problem to solve before we can even throw a bunch of data a sent model and have it learn anything. So to bring this all back, math, statistics and data are fundamentally what construct a machine learning model, and we are hoping to kind of take a different paradigm, or change the paradigm a bit with the sense of smell and being able to learn from less data versus terabytes of data, which is what chatgpt or some of these more intelligent models have been trained
Aaron Moncur:on. You mentioned the data standard for olfaction. What would that look like? I mean, a JPEG I get because I can see a JPEG with my eyes. Or maybe a better way to say that is a computer can show me something that I can perceive, but with olfaction, a computer can't, at least not right now anyway, emit a cent that I can perceive. So what does that data standard look like? Do you think for olfaction? I've been thinking
Kordel France:about this for a long time, and I still don't think I have a. A right answer yet, but here, here's where I think we are. Olfaction is a really weird sense in that there are multiple ways you can detect it. So I mentioned before, you're really just trying to, you're trying to touch a molecule in the air in some way to figure out what it is. Now you can, you can react with it chemically. If you, if you if the sensor touches a chemical, it will create a chemical reaction that sends a voltage back to the computer. That's one way so you can also hear it. The the quantum vibration frequencies for molecules can actually be detected by by different by different different sensors for different receptors. It's called the vibration theory of olfaction, or you can see it. So if you send different wavelengths of light out, they will refract in certain ways that allow you to determine what chemicals are present. So there are at least three different ways you can see, you can touch or you can hear, chemicals in the air for olfaction. And so each one of those has a different data. Standard audio is going to have frequency spectrograms. Right sight is going to have images, right if you if you get detected with infrared or some sort of light wavelength, and then chemical reactions come forth in time series, or they can come forth, and also spectrograms. So if I had to hedge my bets right now, I would think that olfaction is probably going to look something like either these very large graphs, or they're going to look like spectrograms, moving forward, multi, multi spectral, multi time series spectrograms. And that's, that's, that is what I think will be the data standard for smell. So I guess we'll see if time will approve of that or not.
Aaron Moncur:Yeah, I'm thinking about Elon Musk and neuralink right now. Do you envision a future ever where you've got this chip in your brain, and maybe there are some external probes or sensors as well, and people who have lost their sense of smell. Somehow, maybe it's COVID, I don't know. Maybe it's whatever birth defect, something, but they don't have a an olfactory sense where the bionic man basically people can smell again, with with some kind of brain implant that detects whatever that digital standard becomes in the future?
Kordel France:Yes, absolutely. I think we'll be able to have a nose prosthetic, if you will, that allows you to gain your sense of smell back if you've lost it. There's an interesting story that a friend was telling me the other day on Amazon, there was a candle maker who saw a sudden drop in its reviews for scent, and they they couldn't figure out why. Like, their manufacturing processes hadn't changed. They were doing the same quality control methods, and the camera or the candles were being having a lot of complaints by customers saying this, the scented degraded. They couldn't smell them anymore. And it took one statistician. Actually wasn't even a statistician. It was someone who wasn't even familiar with statistics. Familiar with statistics to take a step back and say, okay, when did the reviews start degrading? Well, it was after COVID. What was a consequence of COVID? A lot of people claim they lost their sense of smell. So that is one instance right there where quality control through digital faction sensors probably could have caught that to say, look, the magnitude of chemicals being emitted from this candle as it's burned has not changed. There's something on the human side and this is systemic, right? So it's that's, I always recall that example because it's a really good exclamation point on why digital faction sensors are necessary. But I mean, if they can be recovered, right? I think most of us recovered our sense of smell as you've lost it during COVID, but it is something that's going to be mediated in the future, because some people do permanently lose it or have issues at birth.
Aaron Moncur:Are there sensors right now? I mean, let's say your your candle maker, example. Are there any quality, quality control, olfactions, technologies that are used right now, or are they just kind of relying on people to Yeah, it
Kordel France:smells about right? Based on my research there, there's so there are both. But in the perfume industry, and I'm sure it's the same in the candle industry, or any other sort of perfumery company, they actually have what are called Master perfumers, or, I think that's the actual technical, technical term they give to them. But they are trained to quality control the sense of perfume. So if, for example, you're you're producing Pradas, new perfume that they're putting out, you will have somebody that's been trained to understand and smell exactly what the optimal Prada perfume will be like when it comes off the line. And there's this whole process, which is kind of funny. They smell it, they clear their nose with another, with another sense, because the chemical reaction resets your nose, and then they smell again. And so they sample that to quality control. Now there are, there are digital sensors that actually. Do quality control, but the tried and true, ground truth, way of performing quality control is through humans in the perfume industry, based off of what I've what I've observed and what I've researched and what's been spoken to me by experts in the field. And I find that extremely interesting, because you would never, you would never expect a vision problem to be handled by a human. I mean, they're going mean, they're going to come in and help quality control, but a lot of time that's automated through cameras or or some sort of high speed imagery. So I found that pretty fascinating.
Aaron Moncur:That is fascinating. That's the right word to use, fascinating, and it reminds me of sexing chickens. Have you ever heard about that? I haven't. I'm pretty sure this is not apocryphal, because I've heard it from multiple independent sources, the same thing. But apparently there is a process for sexing chickens whereby a human and this, honestly, this probably has been taken care of by AI at this point, but in the past, to determine if your your baby chicks are male or female, it was people that would would look at them, and there was the technicians doing this, this sexing work. Could not tell you. These are the specific traits or characteristics that I see that, tell me this is a male or a female chick. All they could do was, was look at enough of them, and you know, they're training machine learning in a way, right their own brain, really. And over time, with a coach there to tell them, Oh, this one is male, this one is female. Eventually, their brains would see a pattern that they couldn't really vocalize, but they would know that, yeah, okay, this one's male, this is female, but they didn't really know. You know how they knew that? And so there's this, like profession of sexing chickens, and it just that's what it reminded me of when you're talking about these, what did you call them? Olfactory experts,
Kordel France:or Master perfumists, perfumers, yeah, Master perfume, yeah.
Aaron Moncur:Fascinating, fascinating. Okay, that's well, oh, go ahead, please. No,
Kordel France:it's a really interesting segue. Really interesting point you bring up because as I read more about how these, these uh, judges, these master perfumery judges, actually get the job and actually distinguish traits for different perfumes. It's not exactly evident what creates the sense of smell for fruit, like, how do you smell something and it's fruity, or it's Woody, or it's musky? They actually, there's not a lot of research. There's, well, there's a lot of research, but there's not a definitive way to understand what molecular compounds exhibit fruity smell. We know they do, but interest it's not down to the structure. Some argue it's down to the vibrational frequencies. Some argue that it's down to the distribution of weight in the molecule. Some argue it's down to the difference between electrical orbits or electron orbits. And so there's all these different theories. And on top of that, what smells fruity to me is maybe not going to smell fruity to you because of some of the issues we just discussed. And so smell is so interesting and high dimensional and that it's very hard to have like an objective point of view on what a scent, what a olfactory data sample actually smells like and so I think that further paints the picture, or further paints the emphasis rather, for the need for electronic faction sensors. Yeah,
Aaron Moncur:yeah, that's great. Well, let me take a very short break here and share with the listeners that the being an engineer podcast is brought to you by pipeline design and engineering, where we don't design pipelines, but we do help companies develop advanced manufacturing processes, automated machines and custom fixtures, complemented with product design and R D services. Learn more at teen pipeline.us The podcast is also sponsored by the wave, an online platform of free tools, education and community for engineers. Learn more at the wave dot engineer, today, we're speaking with Cordell France. So Cordell, what are some of the most significant challenges or hurdles right now, specifically in the niche of olfaction. Ai,
Kordel France:there are several. The most significant problem is probably the one I just mentioned previously, where it's not exactly 100% sure on. Nobody's really 100% sure on what chemical properties actually manifest this particular smell. And it really comes down to the quantum level, which is very difficult to measure. On top of that, the lack of data standard. And then furthermore, the initial conditions of any air sample largely influence the actual the way that that air sample behaves over time. And. And so you kind of look at, if you're familiar with chaos theory, right? It's very, very like the butterfly effect. Very small perturbations give very large differences in outcome, and that creates very hard problems for anything aerodynamics. If you talk to any aerodynamicist, it's, it's very hard to model any computational fluid dynamics problem on an aircraft or missile or or submarine or anything because of these kind of chaotic properties and the sensitivity to initial conditions that are just inherent in any environmental or air problem. And you really have to model that in order to detect some chemical within the air. There's no shortage of data available in the air around us, but being able to not only detect but also ground your assumptions in the conditions of the air around you is an extremely hard problem to come across right now, because if I elevate I might detect six different compounds based on the sensors that are giving your response right now, but then I raise the humidity by 20% and Now it looks like those compounds are gone, or I'm detecting a different series of compounds. And so trying to figure out, how do you compensate for our environmental conditions? How do you properly validate your assumptions? What assumptions are critical in order to actually make a software model work? And then on top of that, how do I de noise the data as it's coming through? Because it's always very noisy. So that really tips the hat to the effect of software, machine learning and very complex filters to extract the signal from the noise, if you will. If
Aaron Moncur:you could spend the next five years of your life working on the element or area of olfactory AI you think would have the biggest impact on the world, and you know, all expenses covered, you just got to choose where you work for the next five years. What would that be?
Kordel France:Navigation or aerospace? In my opinion, I think being able to reliably navigate by scent, or did we reliably follow a scent like a dog can and and triangulate the source of the target compound is going to be invaluable in several applications. Because if you're paying attention to the humanoid robotics industry right now, you'll notice the boom of all these companies that are getting billion, literally billions of dollars in investment to build humanoid robots for various functions. And they have eyes, they have ears, they can talk to you, but they're lacking the sense of smell, and you can imagine the applications and how useful it is for the sense of smell to be on a humanoid robot as it goes into service work and all the other things that they plan for those the aspect of aerospace is interesting, because there are a lot of instances where problems Within aircraft can be identified by being able to detect chemicals that are emitted or fuel combustion or fuel leaks. And then on top of that, if you go to the space arena, there are a lot of instances where a big machine called the gas chromatography mass spectrometry machine is used to analyze soil samples or matter samples from other planets to detect signs of organic matter or life, and those machines are extremely large, extremely heavy, extremely costly, very sensitive to the vibrations and the forces of a space launch, and to be able to compress that technology and put it in the palm of your hand, or use a different technology that has an equivalent order of magnitude or equivalent functionality that would solve a lot of problems within the aerospace industry. So I think the navigation and aerospace are the two biggest industries and applications that I would personally work on if there were no one was barred.
Aaron Moncur:Yeah, why do you think that olfaction has not received the same prestige or attention or focus that some of the other senses have in AI, a
Kordel France:lot of it is, in my opinion, and there are several different opinions, but in my opinion, the biggest reasons why it hasn't received as much attention is because There's a non agreed upon data standard that makes things makes things very hard. But I don't think that. I think we're a bit distracted right now with all the other cool things going on. Ai, right? Everyone who's played with chat GPT understands how cool it is and how interesting it is, and how the problems it can solve, and it's, it's, we're very blown away. And that aspect of artificial intelligence, the language part, the audio part, the speech part, is receiving a lot of investment because of that breakthrough that occurred. And I think that if we can make a similar breakthrough in olfaction, we'll see that there are an equivalent amount of problems that can be solved in applications similar to what we just saw with chat GPT. Now, in order to get that to happen, we've got to bring things out of the lab. There's a lot of really interesting research that occurs in the lab, but because of some of the problems I just mentioned, with aerodynamics, it's very difficult to replicate in reality, in a consumer setting, and so being able to I. If I had if I could speak to a group of aerodynamicists and just say, Please help solve this problem. I would hope that somebody would give them a million dollars each to just work on olfaction for a year and just solve a lot of the problems, to help get some of these technologies out of the lab. And in reality, because the aerodynamics are a huge problem and a huge barrier to entry for any olfaction sensor. So I guess, yeah, I guess it comes down to that the aerodynamics.
Aaron Moncur:What kinds of challenges are there with integrating the AI into into mechanical systems, you know, getting it into hardware.
Kordel France:That is a really we're at a really interesting point in time right now, because we're finally seeing hardware and AI Mario. In my mind, I've always seen AI as a psychology of a robot and the hardware as the actual physical beam of a robot. And anyone who is works in hardware software will understand that software gets 10 times harder, if not more, when you start integrating it with hardware, because there are all these different things that you've got to compensate for, you got to test for and calibrate. And so the hardest thing, in my opinion, in my experience, and I built several products with teams that both use hardware and software, is being able to accurately quantify your tests early. So having good tests early that basically allow you to assess the functionality, a very singular functionality of the product, and make sure that it's bulletproof, is something that we've gotten a lot out of in my career. What I mean by that is, a lot of times when you're building a product, you you skip some of the early steps because you might be unsure of the direction, or you need to get a product out quickly, or you're you're subject to all these other these other conditions. And so, you know, testing specific functions aren't important, right? You just want to test the product and make sure the product itself works, but the product itself is a function. Is a series of functions, and there's several different features in there that if one goes wrong, they have this cataclysmic effect on the other. It's a small snowballing effect. And so if you have a very bulletproof test strategy that can automate the functionality of the product and test everything early on as it's developed, you do yourself a huge favor going down the road, and that couldn't be more true than olfaction, because the sensors for olfaction are so sensitive for to material properties and software properties, and making sure that the actual spacing and placing components is not inducing more noise and electrical noise than it would be otherwise. So you have to compensate for these things. And very small, very, very small changes in tolerance have a magnitude, a huge magnitude in effect. And so you really, if you have a really good hardware person and a really good software person that understands the subtleties and testing for both of their respective disciplines, they can make a huge difference on a product development team, especially in a faction. I'm
Aaron Moncur:going to ask you a question that probably falls outside of your area of expertise, but when are we going to have fully autonomous vehicles?
Kordel France:I think within I so I told, I told my wife this the other days. I don't think my kids are going to have to learn how to drive. I think they'll, they'll be given the opportunity to, but it'll be like a motorbike or riding a horse. It's like, yeah, go get your license if you want. You don't need it. I think it'll just be able to recreate, yeah, recreation. So I think, I think within 10 years, it will be like commodity, and it will be, it'll be like a smartphone. It'll be something we don't even think about anymore. But
Aaron Moncur:10 years that's not too far away. No, no, it's
Kordel France:not. I, I really. I mean, you see that way most got they've got autonomous vehicles out various cities. Tesla's just made another announcement. They seem to make one every year that autonomous vehicles are coming the next year,
Aaron Moncur:so to sometime in the next decade, one of Elon's predictions will be correct about his fully self driving vehicle, yeah,
Kordel France:yeah, I agree. It's I had a Tesla and I really liked it, and I really enjoyed seeing the software features that came out in autonomy. But to say it was full self driving is a huge overstatement. And yeah, that definitely we should lose that term in those vehicles until they really are what they are, but they're not full self driving yet.
Aaron Moncur:Yeah. What's your opinion? There's a debate Tesla, specifically, Elon Musk, I think a lot of the engineers there disagree with this, or at least have in the past. He wants just vision like no sensors, right? What's your opinion on that? Do you think that ultimately it's going to end up being a combination of vision and sensors, or is vision truly enough?
Kordel France:Well, in my opinion, I think I think cameras, or just day color cameras, are enough to get human level capability and human level driving. Now I would argue that you want vehicles safer than a human so. Go out like if being able to go 85 miles an hour in a fog, vision is not gonna be able to help you get there, right? You're gonna have to use LIDAR or radar or something that that can see through that because a human I'm not comfortable going 85 miles an hour through a foggy road. Technically, you could, but you've got to be able to communicate to other vehicles and see how far away am I. So there's some signal communication that needs to happen there. It's not just, it's not just cameras that are going to give us super human driving capabilities, but I think they'll get us to human driving capabilities.
Aaron Moncur:That's an important distinction. Yeah, cameras will, will maybe get us to human level driving but really, we'd like to go beyond that
Kordel France:and and to caveat that you need a lot of compute. I was just reading an article earlier this morning where they said that the hardware they thought was going to get them to self driving for Tesla, with what they call hardware three is not going to have a level of compute that they that they think that they're going to need to actually get to full self driving. So they had to have even more compute and more chip design and more sensor optimization. So vision is one part of the problem. But if you put 20 cameras on a vehicle, I would argue you still need to have you still need to have a lot of compute to make sense of that data. Otherwise, it doesn't matter what sensor on the vehicle, because you've got a process that merge it all together semantically and stitch the scene together to have a really good situational awareness of what's going on. What is one
Aaron Moncur:thing that you've done or seen done to accelerate the speed of engineering? One
Kordel France:thing that I've done, and it took me a long time to learn this, is to step aside and let smarter people, people that I manage sometimes, actually take hold of the problem that I'm trying to solve. And what I mean by that is when I when I started a couple startups, if you built a startup, you know that you have to do everything, especially if you founded one. And you start by doing everything. And you mean, you're a mechanical, electrical, software engineer all in one, and then you start hiring, and you start growing. And I'm a micromanager by default, because I really care about the problems I'm trying to solve, and I found that by stepping aside and letting some of the people that I hired or that I worked with take hold of the problem that they would teach me and actually solve the problem better than I could, and it took a lot of humility and a lot of growing personally to understand that, But being able to work together as a team has enabled me to solve a lot more problems at scale, from an engineering fashion and relying on my team and having confidence in them, than just being the lone wolf or link solo, which I did at least for the first five years in my career. So with that being said, you have to make sure that you hire smart people and you work with smart people, but if you trust in them and you them, and you give them ownership of the problem, a lot of people take comfort in that, and they'll teach you along the way, and it just creates an incredible team dynamic. But yeah, I think that sounds cliche, but honestly, that's a tough lesson I had to learn in order to become a better engineer. Was to understand where my strengths were and where my weaknesses were, so that I can either bring up those weaknesses with smarter people or just rely on others in order to go faster and build faster.
Aaron Moncur:That's a terrific and very insightful answer, building on those that lesson learned. If, if you went back in time and visited yourself at the very beginning of your career, what advice would you give your younger self? Oh, wow, there's
Kordel France:a lot. But if I could compress it into just a few seconds worth of advice. One of it, the first thing would be to start working on the sense of smell now very early, and to start moving that industry forward as much as I could. And the second thing would be to be very careful about investment and investors in an idea. If you start a startup, you are, by default, typically looking for investors. And I feel like media, pop culture, kind of glorifies that, but you have to keep in mind that anyone buying into your idea also wants control the idea. And I've had a couple instances where the startup that you think you found and the direction you think you're taking ends up not going the direction you want, because the people who have given you money, and rightly so, should have a say in what you're in, what the direction you want to go, but the ship kind of goes in a different direction than you want. So I would say be very careful about taking on investment and making sure that you properly vet investors and in any idea or startup that you're that you're building, yeah,
Aaron Moncur:very insightful. Sounds like a hard one from from experience. All right, I think we'll, we'll end there. Cordell, this has been really a wonderful conversation, super interesting. I have never really learned anything about olfactory AI. I learned a lot today about the challenges where we are, where we're, where we're going, and what a reassuring feeling it is to know that there is. Super smart people like you out there who are investing their time and resources into bringing us into that future, that that brings us olfaction. I guess before we go, is there anything else that you think we should hit on, any, any topics that we should discuss before ending today?
Kordel France:No, the only parting shot I'll make is I hope that there are more investments and innovation and research and a lot more smart people that go into the field of olfaction to try to raise that industry up to the potential that it has. And I hope that I can make a lasting impact in the field, and I intend to. And yeah, I hope that if you're interested in the field, please follow me on X or LinkedIn. I try to post a lot about olfaction and the interesting applications of it, state of the art, of the technology, to try to solicit more insight and solicit more excitement for the industry. But yeah, I think to all engineers out there, you know, the world moves forward because of us, and largely because of us and others, but engineering is a fantastic discipline, because we're the ones that create the magic in the world. So thank you to your listeners, and thank you to you for hosting an amazing podcast.
Aaron Moncur:I agree, yeah, engineers are the unsung heroes of the world. And who was it? Someone said that magic is just just technology that we don't yet understand, right? So in a very real sense, I think you're correct that engineers are bringing magic into the world.
Kordel France:I agree. Well, thank
Aaron Moncur:you so much for for being on the show today. Cordell, really appreciate you sharing your time with us.
Kordel France:Thank you, Aaron and to your listeners.
Aaron Moncur:I'm Aaron Moncur, founder of pipeline design and engineering. If you liked what you heard today, please share the episode to learn how your team can leverage our team's expertise developing advanced manufacturing processes, automated machines and custom fixtures, complemented with product design and R D services. Visit us at Team pipeline.us. To join a vibrant community of engineers online. Visit the wave. Dot, engineer, thank you for listening. You.