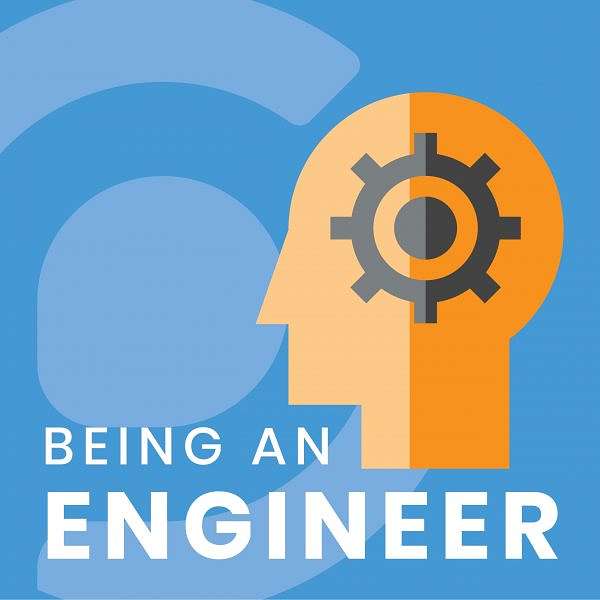
Being an Engineer
Being an Engineer
S4E43 Kevin Surace | How To Use AI For Engineering
As an AI Keynote speaker & disruptive innovation specialist, Kevin Surace helps companies navigate the dynamic landscape of AI-driven transformation. A few of Kevin’s accomplishments include the following:
- Recipient of INC Magazine's Entrepreneur of the Year (2009)
- Built multiple startups from ground zero to $1B+ valuations
- Awarded 94 worldwide patents, including 32 in the US
Join us on this episode to hear how engineers can leverage AI to improve their work and accelerate their careers.
https://appvance.ai/
Aaron Moncur, host
🚀 Join Us at PDX 2025! 🚀
PDX 2025 is the Product Development Expo designed for engineers who want hands-on training from industry experts. PDX focuses on practical skill-building, cutting-edge tools, and real-world solutions.
📅 October 21-22, 2025
📍 Mesa Convention Center, AZ
🔗 https://reg.eventmobi.com/product-development-expo-2025
Click here to learn more about simulation solutions from Simutech Group.
About Being An Engineer
The Being An Engineer podcast is a repository for industry knowledge and a tool through which engineers learn about and connect with relevant companies, technologies, people resources, and opportunities. We feature successful mechanical engineers and interview engineers who are passionate about their work and who made a great impact on the engineering community.
The Being An Engineer podcast is brought to you by Pipeline Design & Engineering. Pipeline partners with medical & other device engineering teams who need turnkey equipment such as cycle test machines, custom test fixtures, automation equipment, assembly jigs, inspection stations and more. You can find us on the web at www.teampipeline.us
Where do engineers and designers at companies like BMW, NASA, and Bosch get custom manufactured parts fast xometry with xometry Anyone with a 3d model can get pricing and order parts thanks to xometry is instant quoting engine X has dozens of manufacturing processes like CNC machining, sheet cutting and fabrication, 3d printing, injection molding and more along with hundreds of materials all in a matter of seconds. Check out xometry today@xometry.com That's xometry.com xometry were big ideas are built Hello, and welcome to another exciting episode of The being an engineer Podcast. Today we're speaking with Kevin Surace as an AI keynote speaker and disruptive innovation specialist. Kevin helps companies navigate the dynamic landscape of AI driven transformation. A few of Kevin's accomplishments include the following recipient of Inc magazine's Entrepreneur of the Year 2009, built multiple startups from ground zero to 1 billion plus valuations and awarded 94 worldwide patents, including 32 in the US, and the list goes on and on. Kevin, welcome to the show. And thank you so much for being with us today.
Kevin Surace:So happy to be here. Thank you for having me.
Aaron Moncur:All right. Well, let's start with what maybe is the softball question, but maybe not. We'll see. The term AI is so ubiquitous these days, especially since chat GPT came out what, what is AI? What are we talking about when we say artificial intelligence?
Kevin Surace:undefinedcertainly scientists and others have worked in the machine learning slash AI space since the 50s. So it's been a long time. 70 years and, and and I think the public just this over the last year, I got excited, because for the first time, they could do something and say, Wow, this is artificially intelligent. It isn't, we'll get into that. But that's what they said. And all of a sudden AI started to mean something. Now, we've hit AR ai ai very much in our regular lives for more than a decade, Facebook as an AI platform always has been in many, many levels, from facial recognition to feeding you the right ads, right. And these were deep learning models, they built them over the last 10 To 12 to 14 years. And, and so that's been in our life. And and of course, it's been in the military life in the FBI is life for decades before that. So we've been using AI and it's been around us even mortgage determination, things like that have been done now for upwards of a decade. But once people could touch it, and do something themselves, they got pretty excited. And then some of them probably got disillusioned and said, Well, it's it's cute, but I don't know what to do with it. The fact of the matter is, all of these are nothing more than mathematical statistical models. And same with shad GPT. It is a large language model as opposed to small language model. And I want you to think of it this way back. Some 40 or 50 years ago, we solved math. What I mean by that is we got calculators, and then we got things like Excel Lotus 123. Before that, then Excel. And since then, nobody had to do long division any longer. Yes, in school in fourth or fifth grade, but after that you don't do it. And you don't do it. Because we have a machine that does that really, really well. It's a great tool for us, right, that amplifies our intelligence. So we've solved math for the human race for a very long time, right for decades. And all of us use those tools. We don't think anything of it. But we didn't solve language, a language tool was much harder to do. And now we have models finally enough compute horsepower in the cloud enough money to solve language. So when we go to Excel, and we say solve this highly complex set of equations, and it does it, we're not shocked, we're happy and it's done. Now we can go to something like Chet GPT, or Bard or others, Obama and solve a language problem simply by asking a question. And it'll solve it. Simply by picking what word comes after the last word. That's all that's going on here. Right? And there's a lot more math than that, it doesn't really matter. But in the end, it is just building sentences and phrases. Great. I'll let me end with this. Let me give you this. Because people always ask, well, you know, I heard a lot and all these other people and they said it's going to be the end of the human race. It's the end of jobs, it's going to kill us. Look, don't hook any of these models to the nuclear arsenal. This is a bad idea. Right? But aside from that, it's not going to kill you. It builds sentences, no different than a calculator, you know, spits out long division.
Aaron Moncur:Well, I heard you say something on another podcast. asked for a presentation somewhere. And the sentiment was I think you're paraphrasing what someone might say. Okay, AI, a rising tide lifts all ships, right. But But oh, well, aren't only the the executives, the people at the very top? Aren't they the only ones we're really going to benefit from this, even if I do keep my job or something like that. And you had an interesting response to that, right? Maybe you could just pick up from here, but what are your thoughts? Yeah, so
Kevin Surace:So look, we have to go back through history. And when you go back through history, there is no question that the owners of companies, you can go back 2000 years, right? Do well, when productivity increases? Fine, nobody's going to argue that point the owners, or the CEOs, or the presidents or whatever the Board of Directors may be whatever, okay, I got that. But it turns out, when people get more productive in their jobs, and companies get more productive, and they produce twice as much output, let's say, with the same number of people, and people are usually your largest cost than almost any business, well, then the company does better. And over time, actually, salaries increase over time, didn't say this year, but over time, and over time, the GDP of the country where those companies are goes up. And when GDP goes up, we know that life gets better. I know not for everyone. So it's this is not meant to be a political statement. It's just, we have a lot of data over 1000s of years, that life is better in high GDP economies. And life is better for the vast majority of US citizens versus someone in in Nigeria, let's say or say, you know, pick some small country that has a very small GDP, and a low per capita income, or you got countries that, you know, don't make a few $100, or maybe$1,000 per person per year. And our per capita in the US is, you know, 30 times that, or 40 times that or something 50 times that. So, yes, a rising tide raises all ships, we want our companies to be more productive, we want to be more productive. And by leveraging AI and these tools, just like finance leveraged Excel, they got more productive. Now the rest of the business can start to get more productive.
Aaron Moncur:Very well said, let's talk about AI in the context of engineering and manufacturing. So how is it changing that landscape right now? And what should we expect within engineering, manufacturing changes over the next maybe five years,
Kevin Surace:so you have a lot of things going on, right? And you have a large language model that actually doesn't know how to do math, well, still use it for that. It's terrible. It'll, it'll be wrong more than 50% of the time, depending on the math problem you give it. It's not meant for math, right? We have Excel for math, that doesn't do math. But it is read everything that humankind ever wrote for the most part. And that's a pretty interesting idea. That means I can ask it, how should I go about designing a part that does x? Now, I'm not saying it's going to be right or wrong. But what it will do is give you five ideas that you never had, what you could stamp that from titanium, I can, how would I do that? And you could ask, how would I do that? Well, there are many companies that do the following process, whatever it is, right? And all of a sudden, it's giving you ideas as if you were writing a novel. And you know, when you're so it's through the design process of manufacturing, right? You're either designing a product, or you're designing a manufacturing process for it, or later, you're in production on that, right. And at all of these points, you can ask the wealth of human knowledge, how might I go about doing this? Now, we've been doing that to some extent with Google for a long time. So this isn't a surprise. But with Google, we would search or Bing. And we would find a bunch of websites. And then we take in that data and we'd read the webs this thing already read all the websites, it read everything that we can find. So it's likely to give you some really interesting answers you wouldn't have thought of. That's what I love using these tools for right now. And that could be that you got a yield issue. And you ask it very, very specifically, not one sentence, but you can get very long prompts. And it will come back with some pretty good ideas. Not all of them are right. But if you're reading a novel, you know how you're looking at the blank screen for a long time, if you're designing something, you're looking at a blank screen, if you've got a problem in manufacturing, sometimes you just stare at the screen, you know that you're only getting 80% yield, but you're scratching your head. And it's so it gets you past the blank screen to start thinking immediately, one minute later. That's a very powerful tool. Lastly, in production, of course, IoT is allowing us to instrument all of our equipment and instrument everything that happens. Quality Inspection, everything we can inspect for better our image recognition is 10 times 100 times what it was just 10 years ago. So the things I can recognize the accuracy the delegatee have that image recognition on the line is unbelievable. So I can find any defect, if I can image it, X ray at CT scan it, you name it, right? It's really fascinating what we can see what we can do and what we can act on. Now, with all of that data,
Aaron Moncur:I have found the same thing to be true, I've found a lot of value in asking Chad GPT, what are 10 ways that I might do ABC, you know, or what were 13 different ways of seeing this particular phrase, it just gives you so many different prompts and ways to think about things which has been super, super useful. There was a TED talk that I watched one of many in which you have appeared. And you said that jobs are a form of human bondage, which I thought was a really interesting way of phrasing that probably a lot of people will agree with that to some extent or another. And how will AI, let's say, you know, a while from now, 30 years, 50 years from now, how is AI going to alleviate that form of human bondage and help us do more of what we want to do and less of what we don't want?
Kevin Surace:Well, you know, many of us love our careers, and many of us don't, it just is what it is that point, but but the point of bondage was that you had to do it, unless you were born into a particular family that you know, had hundreds of millions of dollars, you probably assumed that you had to go to work, everyone had to go to work, why? Well, how do we eat, how to put a roof over our head, we have to go to work. And that is sort of a form of human bondage, we've been, you know, wrapped up and tied up and nine to five, or eight to five, or eight to six, or whatever it is, we have to go work on the line, do the sit at the desk, whatever it is that we do. Well, what AI has the opportunity to do is make us so productive, that the company, the companies that we work for in the organization were for are so much more productive and so much more profitable. That indeed, the four day workweek starts to become quite viable. Because I don't need that fifth day from all of my people, or maybe from any of my people, right, that can happen. And then maybe I only need three days, and maybe only two days, I don't think we get to zero and it's a longer conversation. But but all of a sudden, we're not as trapped that this career five, sometimes six or seven days a week, I actually have to do I can do a lot less of that and spend a lot more time on more creative adventures, whether it's, you know, picking weeds or watching TV or whatever it is. There's a lot of other things in life. You know, we've been known by our careers and this is a very scary thing for most people. And that's why they they are very scared going to lose my job to AI. Well, you'll lose your job to someone else's using AI. That's that's the thing. I don't think you're gonna lose your job to AI most mostly not certainly not in the US today. But you have been known by your career, as I said in the talk that you're referring to, I think, was TEDx Orange County. One of the things I said is that even Joseph, Jesus Father, I don't know his last name, but I know he was a carpenter. Joseph the carpenter. Right. You don't you don't have to be Christian or anything to know Joseph was a carpenter I don't know existed not existed. Joseph the carpenter. That's what he was known for. He couldn't have been known for something else. He's Joseph the carpenter is that you know, the father, essentially of Jesus. So this is really I mean, not the father. But the father. You know what I mean? Sure, yeah. So not to get into religion, but you get the point. So this is really fascinating. So we have to let go over the fact that we walk into parties and say, Hi, what do you do? How about Hi, who are you? Huh?
Aaron Moncur:Now we're talking about identities, not roles. That's a very interesting topic. Yeah. Right. How will AI facilitate that shift between roles and identities? Well,
Kevin Surace:as if AI gives us the intellectual power, all of us have to x, maybe 100 of our brains, right? So we're still managing it, we're still manipulating it, it still can reflect our thoughts. But I can be 100 times more productive. Well, all of a sudden, I've got 100 brain power, but so to you, and so does everyone else. And all of a sudden, my brain isn't so specialist, because everyone's got 100 brain power. And now it's interpersonal skills, communication, it's all kinds of other things that will start to differentiate us even more than they do today.
Aaron Moncur:You referred to this revolution of AI right now as kind of analogous to the renaissance right when painting and sculpting and dance and music and all these things started to really flourish in a way that they just hadn't existed before. And that AI right now is perhaps a second renaissance? What what do you think is on the other side of this second renaissance?
Kevin Surace:Well, I think it's really going to help us rethink and reevaluate our relationship with work in a good way, it is going to open up the opportunity for humans to pursue more artistic endeavors if that's what they want to do. And, you know, there are only a few times in human history. And when we've had an invention that was so profound and impacted most everyone, the wheel was one of them. No question, the wheel changed, transportation, it changed farming, it changed everything. And everyone did at that time, right, the wheel was the AI of the time, I would argue, it was a long time between now and a PC on everyone's desktop or Mac and everyone's desktop but, but there was a time that a computer on your desktop was unheard of, unless you were, you know, a computer programmer in the 60s or 70s, right. And then all of a sudden, by the late 80s, mid to late 80s, everybody got a computer on the desktop, and the secretarial pool assistant pool went away. That was a fundamental change. That means everyone at that moment in time, all of a sudden had to write their own documents people didn't write before they went and talked to someone who would write on a word processor or type it or whatever, there was a fundamental change and actually made everyone substantially more productive. But not at first, it was very painful transition. A lot of people resisted having a computer on the desktop, but eventually, everybody got it. We don't think anything of it today. Okay, next thing that happened, internet, fundamentally changed the way we work. All of a sudden, the way we find customers, the way we interact with customers, the way we interact with our suppliers, everything went to the internet, unbelievable fax machine gone, right, unbelievable. And finally, probably, you know, the smartphone obviously took all of that and made it mobile, so that we could, we could do anything we wanted. Wherever we wanted, we weren't tied to the desktop. So those are big, fundamental societal changes, truly societal changes that impacted our work impacted our life at home. AI is one of those. And that doesn't mean it's going to hit everyone all at once. But over the coming weeks, months and years, it will impact you at home, it will impact you at work, it's going to change your relationship with the way you work and the things you do. We will all be robot overlords, even on an assembly line. And remember, fundamentally, assembly line robots go at a much slower pace than we can do in software. Because it's solenoids, and motors. And they're built the same as they were 100 years ago, they don't change much. So we can only make those things move so fast. But they're getting much smarter in the coming down in costs. So the things we can do even robotically are going to be dramatically impact over the next five or 10 years with with the kind of vision systems and and artificial intelligence machine learning that we can build. And
Aaron Moncur:that's a great segue into my next question, I'd love to delve into that a little bit more deeply. And this is a very selfish question, because I'm going to talk about my company and see what suggestions you might have for a company like pipeline. So pipeline, we do engineering service, and our focus is on developing new manufacturing processes. And then building custom equipment and industrial automation around those processes to help OEMs increase or improve their if their production. So our work relies heavily on like skilled people who need to actively think and make correct decisions over and over again, until we have a new machine that we send out to a customer. It's It's time consuming and expensive. And is AI at a point right now where it can help a company like ours.
Kevin Surace:AI isn't good at design machinery yet. So you can't even the multimodal models, you can't easily go and design something but you can have help in code for sure. All of the code helpers, codecs and others, one from Microsoft, etc. are showing a 50 6050 to 60% improvement in productivity from coders. And this has been measured over the last two years. That is, that is a big deal, right? That means I've more than doubled my capacity to code with the same number of people highly measured, well understood, very scientific study. That is amazing. So all of this equipment has code today. So there clearly is code that has to be written. And in many cases, a code helper will help you write some of that code in a variety of languages. So let's start there. The second thing is, I can I can ask any of the major model Those four ideas around solving certain problems. So I'm trying to make something that's you know, bla bla bla bla bla. And I need equipment to drive up the, you know, probably the throughput and lower the labor and do them in parallel all the normal things we do with equipment like this, right? And or improve quality? And what would be its recommendations. Now, you might not take all the recommendations, but I guarantee you, it's going to give you something to think about that you hadn't thought about. Yeah, they'll say, well use a vision system. But this step four, and you go, I never thought about that. That's a pretty good idea. The other eight ideas were terrible, but there was one golden nugget in there that was worth asking the sage, right?
Aaron Moncur:Because like you said, it's gone out and it's read all the things that you would read otherwise on your own and just compiled them and summarized them for
Kevin Surace:you. It's read every patent. I can't I could read every patent but I'd be dead number one. Number two, I couldn't remember them after a while. So. So think, think about that. Now, people will say yeah, but it hallucinates Okay, no, it doesn't hallucinate this anthropomorphizing this, this mathematical model. It has read fact. And it's read fiction. It's read 1000s 10s of 1000s. of novels, as read, as well as websites as well as patents, right? There is junk in there. There's truths in there, and there's false in there. So, so when it comes out, and you get a model to say, I love you, which they've now put guardrails around, they won't let we'll let it do that. It did that not because it knows how to love. But it has read 1000s and 1000s of novels that have sentence structures about love. And it's just repeating the sentence structure about love. And it knows if you say Chechi Beatty, I'm in love with you. The normal response would be either I'm in love with you, or your academy hate you, or whatever it is, right? It's really fascinating. So but that all came from those novels, so you can get wrong information. And that's why a human needs to look at it, and say, What can I glean from this information? What's new to me? So even if I was at your company, one of the first thing I would do is forget the equipment, I would say, How can I work with a large language model to help me grow our business? Through more awareness? And it's gonna have 10 things I can do. blog posts, what you know, the advertising, social media, ads, targeted media, ads, etc, then I go, Well, I don't know how to do any of that. Can you write some blog posts for me? Oh, yes, it can? Can you give me some ads? Yes, it can, including with visuals, you go, Okay, this is helping the business. I haven't decided on new equipment yet. But this is. So yes, you can use it all the way through for suggest, you know, it is an amplifier of your knowledge. And if you get really good at prompts, it'll really be a great amplifier of your knowledge. So you can go in and get very details of designing this piece of equipment. And this is what it's got to do. And this is what I'm happy with. And here's what I'm not happy with. And this is what's worrying, this is the word, what would you do? It'll tell you,
Aaron Moncur:I didn't realize that you could get that detailed with your prompts. Your prompt
Kevin Surace:can be hundreds of words, really wow. In fact, the way we people say, Well, I'm going to take this large language, well, I'm going to train it on my data, well, you probably not actually training the LLM on your data, what you're doing is you're creating customized prompts that are so tight based on your data. So what we do is we embed a specialized prompt system that goes before the LLM. Because we want the LLM to know everything that it knows right, that's it knows all language, but I want it to go out and learn from my own database. So I can have all let's say, all of the text of things I've designed in the past all the equipment, I've designed the path where all of the patents that around that, or whatever it is. And so I can sort of pre prompt and go out and learn from that and come back and say, here are the best resources. And here's the best answers the closest based on what you asked and feed that to the LLM. So what I'm really saying is based on these best responses, which we know are perfect, but are based on this other model, give me your best response. And it'll tune it towards that. And so that's how you're tuning these models to get them to do what you want, by very complex back end sort of prompts that you don't see. Or if you're good at it, you can prompt it that way.
Aaron Moncur:You seem to think a little bit differently than than most people in a very interesting and dynamic way. The question I was going to ask is what's the first thing you think of in the morning but I think a better way to phrase Is it is is. Has that been? Are there things that you do intentionally to train your brain to think out of the box for lack of a better term? Or is it just the result of a long time? Lots of focus having thought about AI for all these years?
Kevin Surace:So on so I'll tell you, so for me, yes, I've been thinking about AI I worked on the first systems that ultimately came Siri and Cortana and all of those right and, and General Motors OnStar. Um, that was back in the late 90s. We invented the first virtual assistant, the AI based virtual assistant portico, my talk magic talk, they had all these different brand names. Her name was Mary, you could ask actually talk to her as Mary should answer is Mary. He very anthropomorphize. So I've been thinking about that, but but I think bigger is this. I, you opened with, I've got some 94, worldwide patents. All of those were me waking up in the morning saying there's a pain point that needs to be solved. Now, I never liked these companies that have a solution to no one's pain point, right? It's a solution without a problem. No one has that problem. It's an interesting solution. I always wanted to focus on real pain points. And and I think when you look at AI, then I look at AI and say, now all the bad things the bad people can do. Yes, we know that. You know, ransomware artists are using it to get your MFA codes and stuff. Of course, they are big, big surprise. But it's more what can it do for real problems, real humanity, and everybody's got all kinds of problems. So when you throw at me, hey, this is my business. And and I wonder how I can help me, you know, I can I can think of a handful of things already. Let's just do it today. And then dig in more, and then we can do more, right? So I help companies think through these things. I don't have all the answers, but I'm always looking for where are your pain points. Hiring is a big pain point for people today. And the first thing they do is say, Well, I'm going to use AI to find more candidates, you can do that. And that's good. But all of your competitors are also trying to find more candidates, and there's a lack of candidates for most roles. And you know, this, I don't even know your company. And I'm gonna bet that at your company, if you want qualified people to do that work, it's pretty hard to find. It's competitive, hard to find, and it's competitive. There's not enough of them. That's why it's competitive. There's not an oversupply. You know, it's interesting because the sadly, the actors are on strike sags on strike, and I think it's 160,000 actors are on strike. And they said, you know, 80% of them don't make something like 10k You're enacting. And so I see that and I go, Well, clearly, there's like 10,000 acting jobs for 160,000 people. That's a field that has a plethora of people to participate. It's not good or bad. It just is. But there's a lot of fields that don't. And there's a worker shortage, and there's going to be a worker shortage. That's where AI can help us close that gap. Because we've got to close the gap. And how do you close the gap get more productive with the people you have? Because you can't, I can't double the size of the company by doubling the number of people because there aren't double the number of people to get can hire them. So it's a pain point. And it's a problem and AI comes the solution. So you know, it's so much positive, I think it's the best time to be alive. Because people before us could never imagine that you could type something or talk to something and boom. You know, here's the most sophisticated answer you've ever seen.
Aaron Moncur:Yeah, it's all well, I guess there are free version, virtual assistant that is no cost. I know that our sales included, we would hire people in the Philippines for a long time because they were so inexpensive. Well, now we have chat GPT, which is largely free and arguably better
Kevin Surace:about both both Absolutely. And and why not use these tools? Again? What I even asked you, hey, does anyone there use Excel? It would sound like I was you know, I don't know living in the Dark Ages. Right? Of course everyone uses Excel. You can hate it or love it. But we all use it. That's it.
Aaron Moncur:Yeah. Right. One of the things I found interesting with chat GPT AI large language models is that I don't remember to use it all the time. I'm not yet in a perfect habit of using it occasionally. I'll think Oh, that's right. Chappie cheat GPT that's the thing I can use it. What are some ways that we can get ourselves into the habit of remembering it? Yeah,
Kevin Surace:what's gonna happen is all of these are going to be built in as assistants in all of our tools and excel in Word. Because you don't need to go to some separate website or application to use something. It should just be in word and it says, you know, I'm typing and he goes, I need some AI help it goes. It's and that's what Microsoft's working on. That will be built in very soon. And you're going to see this just like cool pilot is built in now to the coding tools at that Visual Studio at Microsoft, right? It's just there. And you just start typing and it starts to finish your code for you. Why? Well, it's just there. It's just a tool. It's a handy tool now. It'll be throughout our life. And we'll use it that way. And we won't think it's Chechi BT or it's Lamarr. It's a bard, or it's just whatever they built in and it works and they put the right guardrails, and they, you know, set it up for me. And it's, it's great. Now we're out solving some bigger problems and working with companies solving problems on like in the building code world, which is pretty interesting. Lots of problems there are there's problems around around writing, like writing novels and writing things like that, that that have to be solved. I think the, you know, the strike with the writers is very much Hollywood writers is very much around who's going to use AI? And how are they going to use it? The initial sort of thinking of you can't use AI to write scripts. That's not going to happen, they are going to use AI to write scripts. And now the question is, do you have a writer to managing that AI as the robot overlord? The that is the right answer, of course. But the problem with that is, you probably don't need four writers in the writers room. Yeah, the writing room. Maybe you need one. And I understand that's a problem. But we don't want you know, producers and directors writing using AI to write their own you want an expert at it? Yeah, just like you're an expert at what you do at your company. And you need to manage the AI that will help you, you know, accelerate your brainpower.
Aaron Moncur:We've talked about this a little bit already. And we may have covered all the answers. But how are the most successful engineering and manufacturing companies harnessing the power of AI? We've talked about just prompts for new ideas. Code, for sure is one, are there any others that come to mind? Are those kind of the the forefront of how these companies are using AI these days?
Kevin Surace:Well, certainly it throughout manufacturing was a manufacturer, I think of not just designed for manufacturing, the manufacturing line and AI is all over the place, and more and more vision systems, more and more measurement systems, more and more QA systems is almost I can say this, if a human could do it on the line, a machine could do it, probably much better. Now, it may not be cost effective. But there is there is no human vision system that will outrun a computer vision system today, if properly coded and properly trained, right, right. If I if I build it on a deep neural net, there's no way that that a human can beat that. And we're going we've seen this in medical right now, the FDA does not allow a company to use AI for say, ECG and have the AI report direct to the patient not allowed. The AI is already far more accurate than the best cardiologists in the country. Why say aye? It is seeing nuances in that heart rhythm that you can't see with your eye can't see it. And in fact, it can't tell you why it sees it the AI can't. Because you got all these hidden layers in the neural nets. Right. But but it is better at it than any cardiologist because it's seeing things you can't see with your eyes. That is what but what it does now as it feeds back to the cardiologists, and then the cardiologists can evaluate and decide if they want to tell you and to decide if they agree or decide if they want to run more tests, et cetera. That is how the FDA has been regulating this right now, AI does not get to talk to you. And and they're doing this with X rays, and, you know, all kinds of imaging in healthcare now, because the AI is very good at it. Okay. AI is also very good. Right now, we don't know why in a brain scan at detecting if you are 10 years or 20 years away from dementia. Ha, but we don't know why. And it doesn't know why. Therefore, almost no one should be telling you that. But we know it's right. Because they were able to go back in brain scans earlier right? From people who have dementia, and the AI was able to pick it out where no human could pick it out. And again, hidden layers can't tell you why. But there's something it's seeing at a you know, at a very small level,
Aaron Moncur:right? There are dozens of little nuances it's picking up on and it can't pinpoint every single one of those for you. It doesn't have the right vocabulary or language to describe it.
Kevin Surace:You see what I look at you and you say this, you know, how will you describe me if you saw me walking down the street? How can you pick me out? I would say well, the nose is like this. He's got headphones on and you beard and you know, whatever mustache, okay, so there's things I could describe to you. However, AI isn't looking just at that. It's looking at nuances that it has no English description of. So people say, you know, this is like a blind box and it can't tell me and it's not explainable. It doesn't have any language to explain, you know, node 45 decision point. That's all All it is in, you know, it's a mathematical model of something that's happening, right. So there's no explanation for that would make any sense. That's what's fascinating. But I'm okay with that. I mean, yes, the black box, but I'm okay with that, because it's giving me more data than I had. Yeah, as long as it's accurate. Great. It's not always accurate. But I get to make the final decision.
Aaron Moncur:Is there another evolutionary jump for large language models? Or is what we have right now, that's what we should expect for the next, you know, 1020 years, whatever. So
Kevin Surace:these AI jumps, as you know, have happened about once a decade big ones, right. The last one was the math to do a deep neural net, right? We could we did neural nets for a long time, but they were only a few layers deep. And then we solved the problem, again, a math problem of what happens if we want a neural net that can go 1000s of layers deep. And that got solved in 2012. And once that got solved, all of a sudden, we had neural nets, deep neural nets or deep learning. That's fascinating that that gave us the image recognition that we have today, in the speech recognition, the other things that dramatically improved, and then we plateaued immediately, like very quickly within a year, and then just sat there. And then Google had an entire team at Google had an interesting idea. It was based on language, and language translation. So we were translating up till then we could translate word for word easily, like English to French. And so as I would speak my English words, or type them at that time, it would give me the French equal once that was put out a French sentence. There's only one problem, that sentence in French made absolutely no sense. Because the sentence structure in French is quite different than English. Right? Right. So someone had the great idea of saying, let's not learn word by word, let's learn phrases. And we'll look at an entire phrase. And there's only so many combinations that you know, you start a phrase with a word, and there's could be a million combinations of that phrase. But if we learn the phrase, then we can translate the phrase properly into another language, because languages are really based on sentence structure, not just the words. So that's what they did. Well, once you did that, you had a model that said, if I learned everything, let's say in the English language, I could then based on a set of prompts, guess what word comes after the last word, based on all of these millions and millions trillions actually a phrases that I learned, what a brilliant idea. That was, that was a step. Now, there were lots and lots and lots of other kinds of trials of doing things, none of them worked very well. But by chance, that translational model, transformer model actually worked really well to build a large language model that is really based on phrases, not words, if it was based on words, you couldn't get anything useful, because it wouldn't know what word comes after another one. But because it's it's this transformer model, it does know this word must come after that one. Based on what you prompted me. Does that make sense? That does make sense. That was a big leap. So to answer your question, you never know when the next big leap is, but most of these leaps have been limited by dollars and compute horsepower. Okay. So, you know, we couldn't have had a deep neural net much before 2012, because we didn't have a cloud with enough tensor flows, or GPUs to do one, right? I mean, we would have had to do them very small, and they really only work having millions and millions of inputs. So it kind of came around with Compute horsepower, right? If you looked at object classification, we would use something 3040 more years ago, called SIFT, which is still used today, that could be processed on a pretty small computer even 40 years ago, and be very efficient. It is not perfect, but it's a very efficient algorithm of which we have hundreds of algorithms. So these tend to follow compute horsepower chat GPT came about, because they were willing to raise over a billion dollars in cash and compute horsepower from Microsoft. So no one else could do it. But them and Google and Facebook and then Apple slightly behind it, because it took a billion dollars of computer horsepower to take in and train a model of a trillion phrases, right? And who has a billion dollars to throw around like you couldn't do a startup and say, give me a billion dollars. I'm gonna go train this model. This is a very unique situation, very unique situation. But think about even that is a very heavy lift. And so those models came about because someone had a billion dollars and then Facebook had a billion dollars to throw it and everybody had a billion dollars that would throw at it because it's very important. And so the big four have that right. Microsoft did, they were working on their own they gave up they, you know, basically license open AI work was fascinating. So, that gets you to the next level. So we are not going to see any huge You know, cloud improvement for the next few years were shorter GPUs shorter TensorFlow unit tensor units, right? But three or four or five years out, you weren't five to 10, you know, there'll be an opportunity for compute horsepower to catch up and then surpass. And then there'll be some other algorithm that you say this is now doable, right? If we had had a chat, GPT model, even 10 years ago, we couldn't have processed it, it would have taken 1010 plus years, right. So you couldn't have done it, you can't do anything that takes, you know, if you're training a model, even for a year, that's, that's an intense, immense effort. You need to train models for days or weeks, even months, because you wouldn't know until the year was up if it was any good. Right? So you see how compute horsepower limits us to what we can do interest as we only have so many years in life, and so many dollars, so, weeks or months, but not yours. So you couldn't have done this. And you couldn't have done deep learning models. Even if you had the math 10 years before they were developed. In 2002, you had you had no compute horsepower to do that. There was no cloud, there was no cloud to go to, you would have had to build the hardware yourself. And it would have been several Cray supercomputers. So
Aaron Moncur:purely speculation here. What let's say that we have the the horsepower to crunch all these numbers, the large data models, what do you think might be included in the next evolution of AI?
Kevin Surace:While so we're already doing multimodal models, as you know, and that includes images and text. And those are coming together now where I can throw an image and text and say, What does this mean? Right, very powerful. But look at it this way, the you know, these LLM 's are not data, data sort of AI models, right. So we've had a models that continue to improve, to crunch through immense amounts of data and give us insights into that data. LMS can also do that, but we have good models for those right. So we can we could train a neural net on a deep learning model right on data, and then start to glean insights from that, that is separate from an LLM, an LLM was trained on for lack of a better phrase, the English language and everything it could find on the web, legal, not legal, good, bad fiction fact trained on everything. And so that's sort of the penultimate of language. Now, we might learn how to get better at it. So it's so it's right more than 80 or 90% of the time, it's not always right, as we know, and we're learning how to correct them. There's also some language model degradation that happens, not everyone is sure why we have some suspicions. We've also got some worries about MLMs, creating so much content on the web, that they lose the ability to learn new content from humans, thus, they start regurgitating only their own stuff. And then that that takes a wide model and makes it very, very narrow, because the vast majority of everything was now created by AI teaching. It's now teaching itself, right, right from So yeah, if you think about 10 years, it could be that every blog post is probably initiated by an AI interaction. Therefore, every blog post that a new AI model reads was written maybe by itself. And so things get pretty narrow. That is concerning for people at the edge of society in a variety of ways, whether it's a, you know, a diversity issue, or, or other kinds of things where it's not dead center nor right, so you could end up with a situation that people are not as represented as right in the middle might get more and more and more out of the zeitgeist of the data that's sitting on the web, because the AI keeps writing more and more and more of the center aisle thing. And that's a dangerous danger, you know, for society, etc. No, it's not going to kill us. It's just like, we should be sensitive to the fact that when we create these tools, there are some side effects, not to kill humanity, but in fairness, and bias and
Aaron Moncur:all of those things. Sure, yeah. Well, what are some ways that you personally use AI to be more productive, some of your, you know, top two or three favorite uses.
Kevin Surace:So I'm a keynote speaker, I do 40 to 50 keynotes a year on AI typically AI disruptive innovation, Silicon Valley innovation, but AI chats up to EMS, etc. is the big topic has been for the last year or so. So I use it to help some things in my presentation. And it's an I give it credit, but it's very appropriate because people are there to learn about AI. And so I usually close my presentation with something written by AI and I asked How should I close the presentation to this particular group to this base? than the following things big prop that says, and I say 200 Words or whatever, boom, boom, boom, boom, boom. And frankly, the pros that it gives me are way beyond my English skills. So I am happy to use it and give it credit right now, I smartly prompted it, I'm the person standing there, right? But so I use it for a lot of that. And also, I might be in front of a group, I'm going to pretend it's tree surgeons, right? Just come look at these beautiful trees outside my windows here, pre surgeons, so I'm standing in front three surgeons are no, I'm going to speak to them next week. And they say, Now bring the top 10 things that tree surgeons could use AI for. And I have several in my mind, but I go, Well, let me ask AI, how it should, how they it would think that tree surgeon should use it. It'll come up with, you know, a lot that I already knew, it'll come up with two or three. But you know, SAP analysis versus, you know, via visual, I go, Wow, that's pretty cool. Right? I couldn't have thought of that, because I'm not an expert tree surgeon. And so the application of AI which which, by the way, applied AI is very different than the creation of algorithms. So Google, open AI, others, they have absolute math, you know, experts on creating immense models, incredible models, right? That is truly r&d in AI. I have spent my time applying AI at the state of the art it is, is a different thing. You have to know how it works. You have to know the underpinnings, you have to know its limitations, then you have to say, Do I know enough about this field to figure out where best I can apply it, right? But one of my favorites is just ask AI where it would apply it and you'll find some that are useful and some that aren't. But they're new ideas for you. That will give it to you. Or I'm gonna I know what tree surgeons do. But pretend I didn't even know what a tree surgeon does. I would normally go to Google and start reading about research. But I could just ask Chechi Beatty or another LLM? What does a tree surgeon do? Tell me in 200 words or less? And it will? Yeah. It's one of the books summaries that we used to have in college. The yellow ones Cliff Oh, yes,
Aaron Moncur:this is Cliff Notes, right
Kevin Surace:Cliff Notes. It's like the Cliff Notes. But it's the length of cliff notes that I need. I wrote something today for a talk tomorrow. And I said, just for giggles, in this time, describe this person and, and and describe it in the style of Shakespeare. And there it was in the style of Shakespeare. And it's funny and fun. And I do a lot with visuals. And you know, sometimes I can't think of a visual that I want or I can think of it but I can't draw it myself. Or I look at it and say this will take me a long time manually doing Photoshop because I am a Photoshop person. But now Photoshop has an AI assistant in it. And I say just, you know, take out this background and add this here, boom, you go. I know how to do it would have taken me two hours to make it look good. It took it one minute, I'm done. Right still have know how to use the tool. I've still the robot overlord. But look how much more productive it made me. And that gave me more time to think about the topic and what I want to present and makes me think a better presenter. So you should use it never the every presentation you're going to do. There's opportunities to say how how should I best state this, it's your statement you're prompting with I know what I want to say, don't know how best to say this. How can for for example, I'll give you credit, you know, funny ones, you get a text from someone and it's just beautifully written, it's a text. And you go, Well, I could respond the way I normally do, which is Thank you. Or I'll ask an LLM and take the entire text put it in the LLM and say How should I respond to this person that will make them feel truly loved and special today? And the prose that it uses? Of course it read novels, right? knows, you know, can it can write something that I couldn't ever write myself? I could? No, I often go and change a few words because they said Well that doesn't sound like me or this you know, whatever. But nine I couldn't have come up with it myself couldn't have right couldn't have.
Aaron Moncur:Yeah, I someone sent me an email a little while ago, I was following up on a project that they wanted to do. And they they responded and said, I'm sorry, my hair's been on fire lately. I just haven't had a chance to get to this. And I had the same thought as you just described. I could just respond to this. Thank you write some lame, boring way or let's put this into chat GPT and see what they respond. So I did that and said how can I respond in a clever way? And it's it took my hair's on fire and something something to the effect of good luck with your flamboyant fire party or something like that. Oh, it was hilarious. Yeah. They thought it was funny and I thought it was funny and it was good. Good to
Kevin Surace:you. People can people share could perhaps start using it to communicate in their relationship. Because you can ask advice as if your psychologist was right there with you. You know, hey chat, GBT, my wife or my husband, baba, baba, Yvonne, I'm not sure how to respond, and I don't want to upset them. Notice by last phrase, you're very important, very specific not want to upset them. What should I say? It'll come back with ideas for you that you probably wouldn't have had. Because you know, humans tend to respond, especially in relationships in certain ways that don't always end up where you would hope to end up, they usually end up with a slammed door or something like this. This is just the way humans relate. And this is true at work, too. So how Bescot okay, I had let's say, I have to terminate someone, chat GPT? How best can I handle this? They've been here 12 years, they're one of my good friends, I really don't know what to say, what should I say? It has read hundreds of 1000s of pros, on firing people. And it will actually have some really great ideas on how to handle this terrible situation that no one wants to be on either side of that conversation. You don't want to get fired, and you don't want to do the firing. But it happens every day. Help me. That's
Aaron Moncur:brilliant. I often say that. In terms of an engineers career growth. If you're the absolute smartest person in the room, you have the best technical engineering skills of anyone, you know, top point oh, one percentage of the population, there's only so far you can go in your career if you don't have really great soft skills, communication skills. And so exactly, perhaps one of the ways that engineers can really leverage AI, if your communication skills your EQ is not so great, is to use it for that purpose. So you already got the technical skills, now let's write it with.
Kevin Surace:That's right AI even pay even if they are great, you're not an HR person to even better now, your EQ may be fabulous in your engineering profession. But you know what, as you move up the ladder, you're a manager and a director and a VP, you're hiring people, and you're firing people. And nobody really gave you training to do that, interestingly enough, and you're not an HR expert. And so as the darn thing, you can use it or not use it right? But But what are you doing, you're amplifying your brain power, all of a sudden, you have the power of an HR person for that for that one set of phrases, that that would have spent decades learning how to do that, right? Because Chet GPT knows. And you can say, I need this prose, I need these sentences in the style of the finest, most caring HR person ever to walk on the planet Earth. And it'll do that it'll work hearing.
Aaron Moncur:That's great. Great, and incredible. All right. Well, Kevin, what a great discussion that has been. Thank you so much for joining us today. Let me ask you one more question. And then we'll wrap this up. And maybe this ties into the very end of the podcast anyway. Where can we go to learn more about the cutting edge of AI? How we can use it? How we should use it? I think following you is probably one great idea. I'll just put that out there. What else can we do to keep up to date on the best?
Kevin Surace:Practice? Sure, sure. I'm on LinkedIn, I'm on Twitter, I'm on threads, so I'm easy to find. They're my websites, Kevinsurace.com, that's pretty easy to find. It's just my full name.com. Look, I think AI moves in this kind of technology moves so quickly, that unless you're really in that field, you cannot keep up. You know, you need people around you, you need people to come in and look at your situation, say here's how we apply it here, which I do with many with many companies, right? I've got I've got a number of companies I've worked with the app fans, I'm, I'm a CTO and share it out pants. And that is a software quality technology using AI to find bugs for you. Only certain kinds, like web software, etc. But nevertheless finds the bugs in the software for you. That's pretty amazing. And it uses the software the way humans would and says, Hmm, that doesn't look right, or this didn't come out, right, or that request to the server didn't happen or whatever happens, right? Without writing test scripts, which is what we traditionally did. So the things that we can do, you know, you really need to, you can read all you want, but you'll be overwhelmed, because it's not your number one job, you know, go to Kevin straights.com, you can find me you can, you know, would message me on LinkedIn, which people do all the time. And that's the easiest way to find me and I always publish really cool stuff too. Perfect.
Aaron Moncur:Great. Well, Kevin, thank you so much this podcast. Awesome. We appreciate that. All right. Well, thank you so much for your time today, Kevin. I really appreciate it.
Kevin Surace:Thank you. There's a lot of fun.
Aaron Moncur:I'm Aaron Moncur, founder of pipeline design and engineering. If you liked what you heard Today please share the episode. To learn how your team can leverage our team's expertise developing turnkey equipment, custom fixtures and automated machines and with product design, visit us at Teampipeline.us. Thanks for listening.